Reducing Evaluation Bias in Speech Recognition
Discover how Rev's new multitranscript method reduces bias in speech recognition evaluation, revealing more accurate measurements of AI transcription quality.
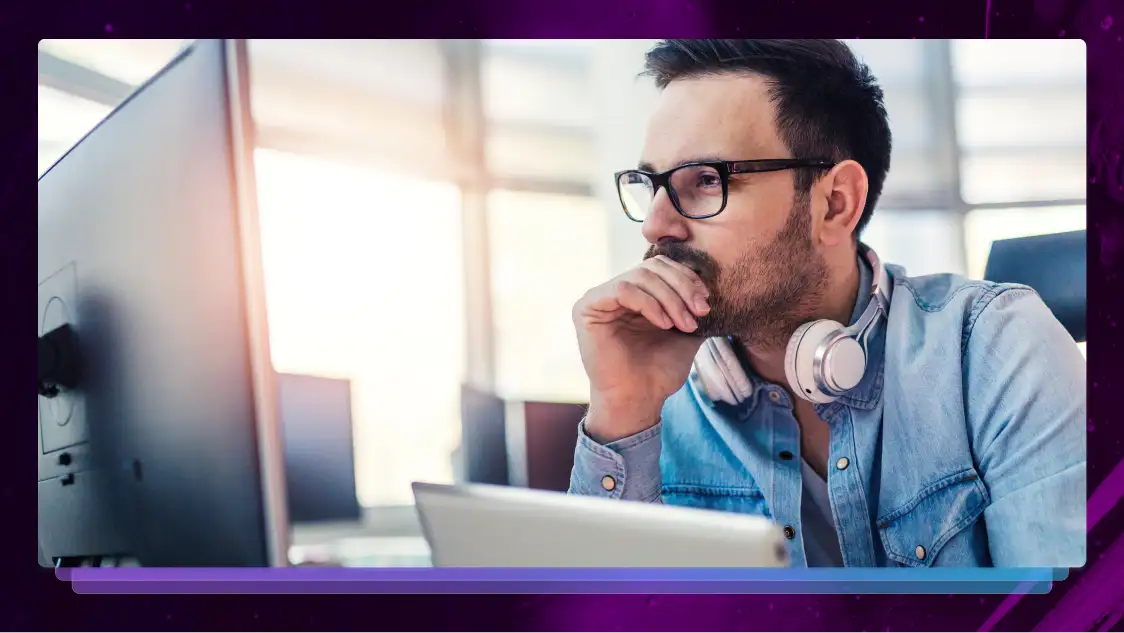
Rev's commitment to accuracy in speech-to-text technology has led to a groundbreaking discovery in how we evaluate AI transcription models. Our latest research reveals that traditional evaluation methods might be missing the mark – and the real accuracy rates could be better than we thought.
We’re constantly evaluating our internal Reverb model, open source models like Whisper and Canary, and speech-to-text systems of other companies to understand where we are and where the community needs to go to make our goal a reality. A big part of this work is ensuring evaluations are as fair and unbiased as possible – in this project, we’ve identified a new way to evaluate that can reduce transcription style bias.
A Tale of Two Transcription Styles
At Rev, we provide two styles of transcripts:
- Verbatim transcripts are created by the transcriptionists writing exactly what they hear, including filler words, stutters, interjections (active listening), and repetitions.
- Non-verbatim transcripts are created by lightly editing for readability without changing the structure or meaning of the speech. These are sometimes called clean transcriptions.
If you’ve ever submitted the same audio through both pipelines, you would find that these choices can result in very different outcomes — that’s not to say that either of these transcripts is wrong but rather is just stylistically different.
Uncovering Hidden Biases in Model Evaluation
When it comes to speech-to-text models, we run into the same situation. Rev’s models are trained to explicitly output verbatim or non-verbatim styles but that doesn’t mean other models can do the same thing. They may be closer to what we consider “verbatim” or “non-verbatim” but they can also be somewhere in-between or maybe even something else entirely. And just like with human transcripts, just because these transcripts are different stylistically, that doesn’t make them wrong.
Up until now, if we wanted to account for style we’ve been limited to evaluating speech-to-text models on either “verbatim” or “non-verbatim” only transcripts. And while it does give us some information, we’re still biasing our evaluation toward our own Rev styles.
Groundbreaking Results From Real-World Testing
We decided to expand upon two existing open source datasets to demonstrate this evaluation bias. Rev16 (podcasts) and Earnings22 (earnings calls from global companies) being verbatim datasets, we produced the corresponding non-verbatim transcripts and compared the word error rate (WER) of our internal model and Open.ai’s Whisper API. As you can see, our Verbatim API does better on the verbatim style transcripts, our Non-Verbatim API does better on the non-verbatim style, and Whisper floats somewhere between.
WER on Rev16
WER on Earnings22 Subset10
The Multitranscript Solution: A New Era of Evaluation
In this release, we provide code to produce fused-transcripts we call “multitranscripts” that allow our evaluation to be more flexible to different stylistic choices. When we use these multitranscripts instead of the single-style transcripts, we find a sizable difference in performance.
Given that all three APIs see a big improvement, it seems like the rate of real errors and not stylistic errors is a lot lower than previously thought!
WER on Rev16
WER on Earnings22 Subset10
Surprisingly, our initial evaluation showed that Rev’s API was better than the OpenAI API by about 20% on average but the new method shows that OpenAI surpassed us by about 15% on Earnings22 Subset10 and remains only slightly behind on Rev16! We’ve only just scratched the surface of this technique and are excited to continue exploring how to improve our evaluations.
Want to dive deeper into the technical details? Check out our full research paper on arXiv.
Heading
Heading 1
Heading 2
Heading 3
Heading 4
Heading 5
Heading 6
Lorem ipsum dolor sit amet, consectetur adipiscing elit, sed do eiusmod tempor incididunt ut labore et dolore magna aliqua. Ut enim ad minim veniam, quis nostrud exercitation ullamco laboris nisi ut aliquip ex ea commodo consequat. Duis aute irure dolor in reprehenderit in voluptate velit esse cillum dolore eu fugiat nulla pariatur.
Block quote
Ordered list
- Item 1
- Item 2
- Item 3
Unordered list
- Item A
- Item B
- Item C
Bold text
Emphasis
Superscript
Subscript
Subscribe to The Rev Blog
Sign up to get Rev content delivered straight to your inbox.